Relighting4D
Relighting4D
: Neural Relightable Human from Videos#
Zhaoxi Chen, Ziwei Liu
NeRF
SMPL
Monocular
Relight
ECCV 2022
FrozenBurning/Relighting4D
[ECCV 2022] Relighting4D: Neural Relightable Human from Videos
Python
271
19
Abstract#
Human relighting is a highly desirable yet challenging task. Existing works either require expensive one-light-at-a-time (OLAT) captured data using light stage or cannot freely change the viewpoints of the rendered body. In this work, we propose a principled framework, Relighting4D, that enables free-viewpoints relighting from only human videos under unknown illuminations. Our key insight is that the spacetime varying geometry and reflectance of the human body can be decomposed as a set of neural fields of normal, occlusion, diffuse, and specular maps. These neural fields are further integrated into reflectance-aware physically based rendering, where each vertex in the neural field absorbs and reflects the light from the environment. The whole framework can be learned from videos in a self-supervised manner, with physically informed priors designed for regularization. Extensive experiments on both real and synthetic datasets demonstrate that our framework is capable of relighting dynamic human actors with free-viewpoints.
PaperApproach#
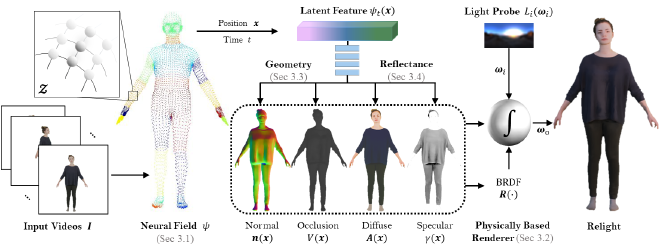
Relighting4D
overview.Results#
Data#
Comparisons#
Performance#
Papers Published @ 2022 - This article is part of a series.
Part 19: This Article