SelfNeRF
SelfNeRF
: Fast Training NeRF for Human from Monocular Self-rotating Video#
Bo Peng, Jun Hu, Jingtao Zhou, Juyong Zhang
NeRF
SMPL
Monocular
arXiv 2022
Abstract#
In this paper, we propose SelfNeRF, an efficient neural radiance field based novel view synthesis method for human performance. Given monocular self-rotating videos of human performers, SelfNeRF can train from scratch and achieve high-fidelity results in about twenty minutes. Some recent works have utilized the neural radiance field for dynamic human reconstruction. However, most of these methods need multi-view inputs and require hours of training, making it still difficult for practical use. To address this challenging problem, we introduce a surface-relative representation based on multi-resolution hash encoding that can greatly improve the training speed and aggregate inter-frame information. Extensive experimental results on several different datasets demonstrate the effectiveness and efficiency of SelfNeRF to challenging monocular videos.
PaperApproach#
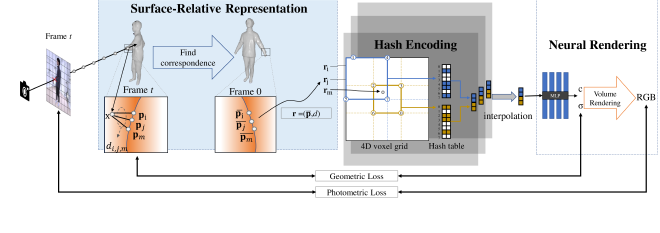
SelfNeRF
overview.Results#
Data#
Comparisons#
Performance#
Papers Published @ 2022 - This article is part of a series.
Part 25: This Article